Research
RNA-Ligand Interaction
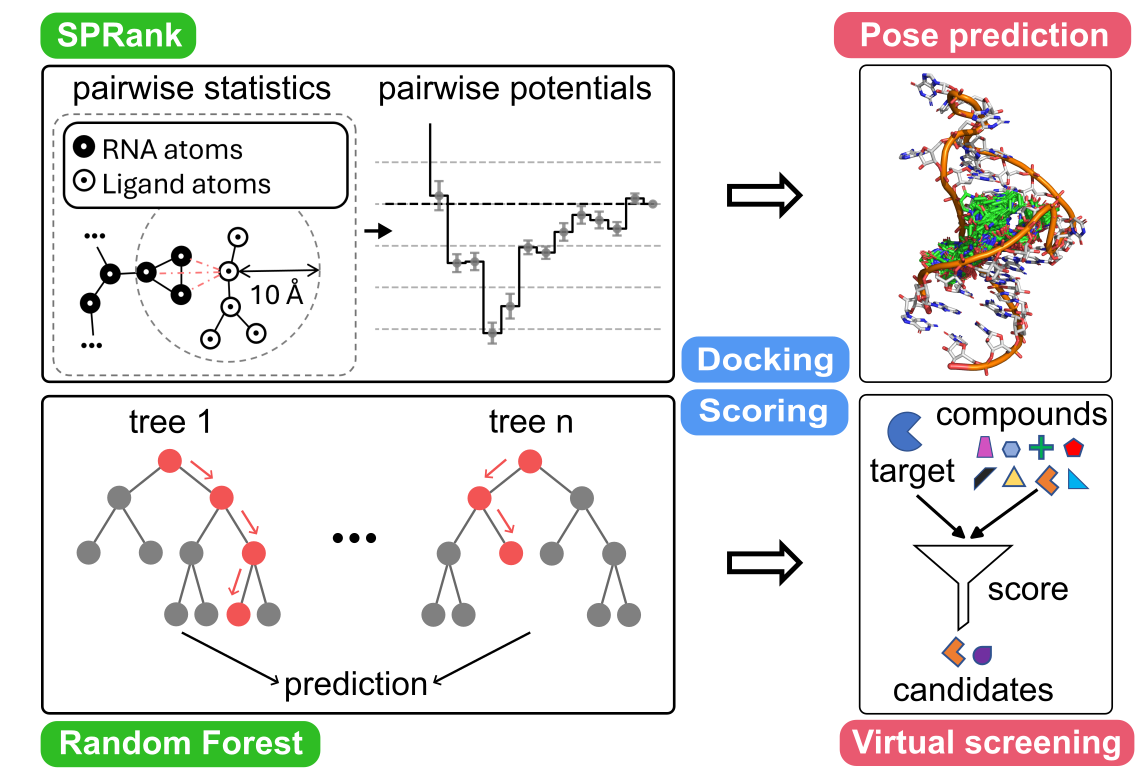
Identifying small molecules that bind RNA targets
can lead to new therapeutics for diseases involving dysregulated RNA functions.
Effective computational screening in this field demands robust docking software
and scoring functions to predict binding modes and enable virtual screening.
Our recent work focus on developing two new tools:
SPRank [1] (https://github.com/Vfold-RNA/SPRank\)
and RLDOCK-V2 [2] (https://github.com/Vfold-RNA/RLDOCK-V2\).
SPRank, a hybrid machine-learning and knowledge-based scoring function,
enhances docking tools by sampling flexible ligands against diverse RNA structures.
Compared to original RLDOCK [3],
RLDOCK-V2 [2] offers an exhaustive sampling of binding sites and an accurate energy scoring.
Both models have shown improved binding mode prediction
and virtual screening across multiple RNA targets such as HIV-1 TAR,
underscoring their potential in RNA-targeted drug discovery.
1. Zhou Y, Jiang YW, Chen SJ.
SPRank - A Knowledge-Based Scoring Function for RNA-Ligand Pose Prediction and Virtual Screening.
Journal of Chemical Theory and Computation,
2024 Aug. doi: 10.1021/acs.jctc.4c00681.
2. Sun LZ, Jiang Y, Zhou Y, Chen SJ.
RLDOCK: a new method for predicting RNA–ligand interactions.
Journal of Chemical Theory and Computation,
2020 Oct. doi: 10.1021/acs.jctc.0c00798.
Metal Ion-RNA Interaction
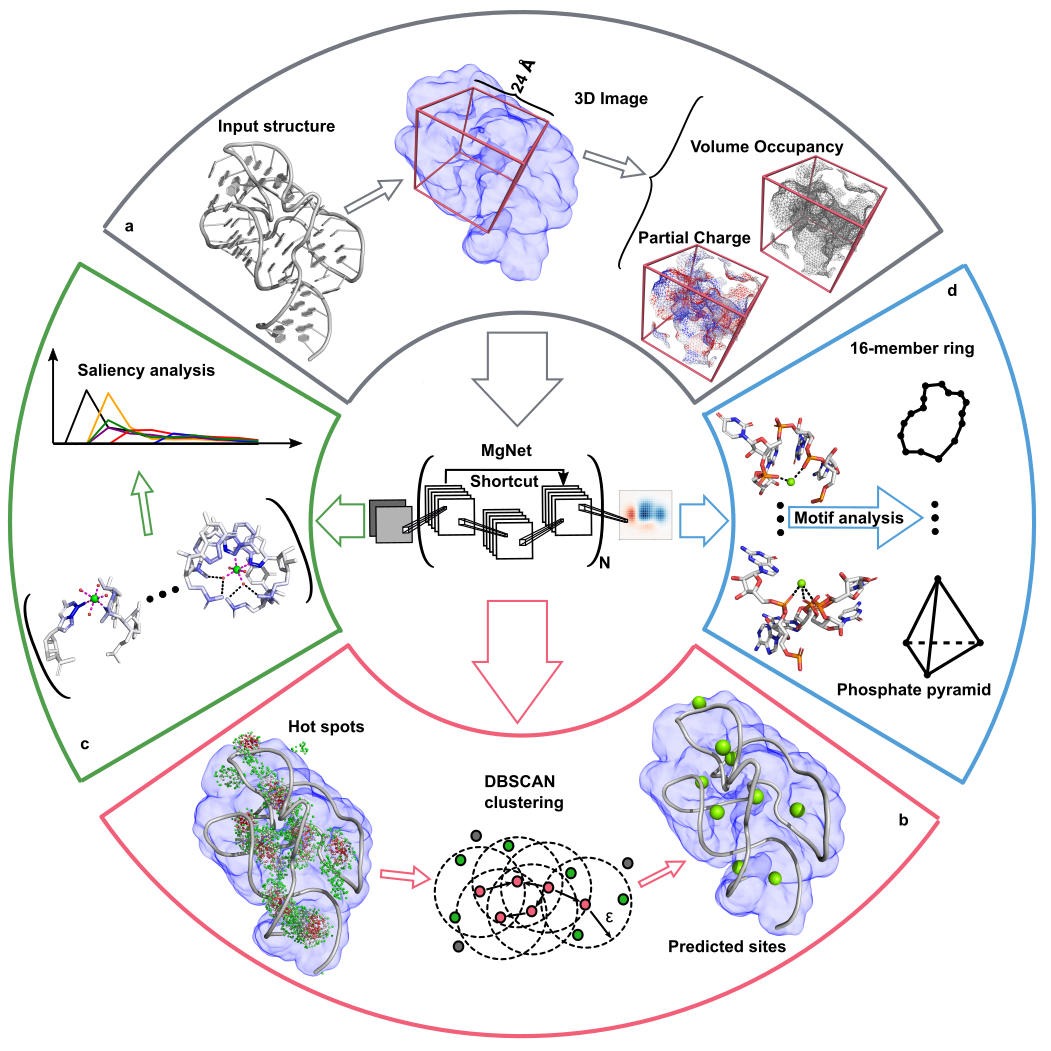
Metal ions are critical for RNA structure and function,
but accurately modeling metal ion (e.g., Mg²⁺) effects remains a major challenge.
Recently, we have created a deep learning method, MgNet,
that predicts Mg
2+ binding sites tightly coordinated with RNA atoms [1]
(https://github.com/Vfold-RNA/MgNet\).
MgNet identifies critical atoms for inner/outer-sphere coordination,
while also uncovering new motifs.
This model can complement X-ray crystallography for precise metal ion site identification.
1. Zhou Y, Chen SJ.
Graph deep learning locates magnesium ions in RNA.
QRB Discovery,
2022 Jan. doi: 10.1017/qrd.2022.17.
©
Yuanzhe Zhou,